Section 14.5 : Lagrange Multipliers
In the previous section we optimized (i.e. found the absolute extrema) a function on a region that contained its boundary. Finding potential optimal points in the interior of the region isn’t too bad in general, all that we needed to do was find the critical points and plug them into the function. However, as we saw in the examples finding potential optimal points on the boundary was often a fairly long and messy process.
In this section we are going to take a look at another way of optimizing a function subject to given constraint(s). The constraint(s) may be the equation(s) that describe the boundary of a region although in this section we won’t concentrate on those types of problems since this method just requires a general constraint and doesn’t really care where the constraint came from.
So, let’s get things set up. We want to optimize (i.e. find the minimum and maximum value of) a function, \(f\left( {x,y,z} \right)\), subject to the constraint \(g\left( {x,y,z} \right) = k\). Again, the constraint may be the equation that describes the boundary of a region or it may not be. The process is actually fairly simple, although the work can still be a little overwhelming at times.
Method of Lagrange Multipliers
- Solve the following system of equations. \[\begin{align*}\nabla f\left( {x,y,z} \right) & = \lambda \,\,\nabla g\left( {x,y,z} \right)\\ g\left( {x,y,z} \right) & = k\end{align*}\]
- Plug in all solutions, \(\left( {x,y,z} \right)\), from the first step into \(f\left( {x,y,z} \right)\) and identify the minimum and maximum values, provided they exist and \(\nabla g \ne \vec{0}\) at the point.
The constant, \(\lambda \), is called the Lagrange Multiplier.
Notice that the system of equations from the method actually has four equations, we just wrote the system in a simpler form. To see this let’s take the first equation and put in the definition of the gradient vector to see what we get.
\[\left\langle {{f_x},{f_y},{f_z}} \right\rangle = \lambda \left\langle {{g_x},{g_y},{g_z}} \right\rangle = \left\langle {\lambda {g_x},\lambda {g_y},\lambda {g_z}} \right\rangle \]In order for these two vectors to be equal the individual components must also be equal. So, we actually have three equations here.
\[{f_x} = \lambda {g_x}\hspace{0.25in}{f_y} = \lambda {g_y}\hspace{0.25in}{f_z} = \lambda {g_z}\]These three equations along with the constraint, \(g\left( {x,y,z} \right) = c\), give four equations with four unknowns \(x\), \(y\), \(z\), and \(\lambda \).
Note as well that if we only have functions of two variables then we won’t have the third component of the gradient and so will only have three equations in three unknowns \(x\), \(y\), and \(\lambda \).
As a final note we also need to be careful with the fact that in some cases minimums and maximums won’t exist even though the method will seem to imply that they do. In every problem we’ll need to make sure that minimums and maximums will exist before we start the problem.
To see a physical justification for the formulas above let’s consider the minimum and maximum value of \(f\left( {x,y} \right) = 8{x^2} - 2y\) subject to the constraint \({x^2} + {y^2} = 1\). In the practice problems for this section (problem #2 to be exact) we will show that minimum value of \(f\left( {x,y} \right)\) is -2 which occurs at \(\left( {0,1} \right)\) and the maximum value of \(f\left( {x,y} \right)\) is 8.125 which occurs at \(\left( { - \frac{{3\sqrt 7 }}{8}, - \frac{1}{8}} \right)\) and \(\left( {\frac{{3\sqrt 7 }}{8}, - \frac{1}{8}} \right)\).
Here is a sketch of the constraint as well as \(f\left( {x.y} \right) = k\) for various values of \(k\).
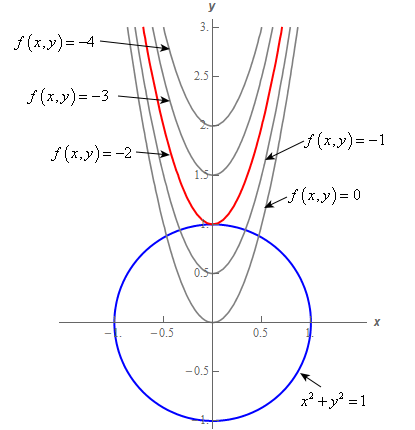
First remember that solutions to the system must be somewhere on the graph of the constraint, \({x^2} + {y^2} = 1\) in this case. Because we are looking for the minimum/maximum value of \(f\left( {x,y} \right)\) this, in turn, means that the location of the minimum/maximum value of \(f\left( {x,y} \right)\), i.e. the point \(\left( {x,y} \right)\), must occur where the graph of \(f\left( {x,y} \right) = k\) intersects the graph of the constraint when \(k\) is either the minimum or maximum value of \(f\left( {x,y} \right)\).
Now, we can see that the graph of \(f\left( {x,y} \right) = - 2\), i.e. the graph of the minimum value of \(f\left( {x,y} \right)\), just touches the graph of the constraint at \(\left( {0,1} \right)\). In fact, the two graphs at that point are tangent.
If the two graphs are tangent at that point then their normal vectors must be parallel, i.e. the two normal vectors must be scalar multiples of each other. Mathematically, this means,
\[\nabla f\left( {x,y,z} \right) = \lambda \,\,\nabla g\left( {x,y,z} \right)\]for some scalar \(\lambda \) and this is exactly the first equation in the system we need to solve in the method.
Note as well that if \(k\) is smaller than the minimum value of \(f\left( {x,y} \right)\) the graph of \(f\left( {x,y} \right) = k\) doesn’t intersect the graph of the constraint and so it is not possible for the function to take that value of \(k\) at a point that will satisfy the constraint.
Likewise, if \(k\) is larger than the minimum value of \(f\left( {x,y} \right)\) the graph of \(f\left( {x,y} \right) = k\) will intersect the graph of the constraint but the two graphs are not tangent at the intersection point(s). This means that the method will not find those intersection points as we solve the system of equations.
Next, the graph below shows a different set of values of \(k\). In this case, the values of \(k\) include the maximum value of \(f\left( {x,y} \right)\) as well as a few values on either side of the maximum value.
Again, we can see that the graph of \(f\left( {x,y} \right) = 8.125\) will just touch the graph of the constraint at two points. This is a good thing as we know the solution does say that it should occur at two points. Also note that at those points again the graph of \(f\left( {x,y} \right) = 8.125\)and the constraint are tangent and so, just as with the minimum values, the normal vectors must be parallel at these points.
Likewise, for value of \(k\) greater than 8.125 the graph of \(f\left( {x,y} \right) = k\) does not intersect the graph of the constraint and so it will not be possible for \(f\left( {x,y} \right)\) to take on those larger values at points that are on the constraint.
Also, for values of \(k\) less than 8.125 the graph of \(f\left( {x,y} \right) = k\) does intersect the graph of the constraint but will not be tangent at the intersection points and so again the method will not produce these intersection points as we solve the system of equations.

So, with these graphs we’ve seen that the minimum/maximum values of \(f\left( {x,y} \right)\) will come where the graph of \(f\left( {x,y} \right) = k\) and the graph of the constraint are tangent and so their normal vectors are parallel. Also, because the point must occur on the constraint itself. In other words, the system of equations we need to solve to determine the minimum/maximum value of \(f\left( {x,y} \right)\) are exactly those given in the above when we introduced the method.
Note that the physical justification above was done for a two dimensional system but the same justification can be done in higher dimensions. The difference is that in higher dimensions we won’t be working with curves. For example, in three dimensions we would be working with surfaces. However, the same ideas will still hold. At the points that give minimum and maximum value(s) of the surfaces would be parallel and so the normal vectors would also be parallel.
Let’s work a couple of examples.
Before we start the process here note that we also saw a way to solve this kind of problem in Calculus I, except in those problems we required a condition that related one of the sides of the box to the other sides so that we could get down to a volume and surface area function that only involved two variables. We no longer need this condition for these problems.
Now, let’s get on to solving the problem. We first need to identify the function that we’re going to optimize as well as the constraint. Let’s set the length of the box to be \(x\), the width of the box to be \(y\) and the height of the box to be \(z\). Let’s also note that because we’re dealing with the dimensions of a box it is safe to assume that \(x\), \(y\), and \(z\) are all positive quantities.
We want to find the largest volume and so the function that we want to optimize is given by,
\[f\left( {x,y,z} \right) = xyz\]Next, we know that the surface area of the box must be a constant 64. So this is the constraint. The surface area of a box is simply the sum of the areas of each of the sides so the constraint is given by,
\[2xy + 2xz + 2yz = 64\hspace{0.5in} \Rightarrow \hspace{0.5in}xy + xz + yz = 32\]Note that we divided the constraint by 2 to simplify the equation a little. Also, we get the function \(g\left( {x,y,z} \right)\) from this.
\[g\left( {x,y,z} \right) = xy + xz + yz\]The function itself, \(f\left( {x,y,z} \right) = xyz\) will clearly have neither minimums or maximums unless we put some restrictions on the variables. The only real restriction that we’ve got is that all the variables must be positive. This, of course, instantly means that the function does have a minimum, zero, even though this is a silly value as it also means we pretty much don’t have a box. It does however mean that we know the minimum of \(f\left( {x,y,z} \right)\) does exist.
So, let’s now see if \(f\left( {x,y,z} \right)\) will have a maximum. Clearly, hopefully, \(f\left( {x,y,z} \right)\) will not have a maximum if all the variables are allowed to increase without bound. That however, can’t happen because of the constraint,
\[xy + xz + yz = 32\]Here we’ve got the sum of three positive numbers (remember that we \(x\), \(y\), and \(z\) are positive because we are working with a box) and the sum must equal 32. So, if one of the variables gets very large, say \(x\), then because each of the products must be less than 32 both \(y\) and \(z\) must be very small to make sure the first two terms are less than 32. So, there is no way for all the variables to increase without bound and so it should make some sense that the function, \(f\left( {x,y,z} \right) = xyz\), will have a maximum.
This is not an exact proof that \(f\left( {x,y,z} \right)\) will have a maximum but it should help to visualize that \(f\left( {x,y,z} \right)\) should have a maximum value as long as it is subject to the constraint.
Here are the four equations that we need to solve.
\[\begin{equation}yz = \lambda \left( {y + z} \right)\hspace{0.75in}\left( {{f_x} = \lambda {g_x}} \right) \label{eq:eq1}\end{equation}\] \[\begin{equation}xz = \lambda \left( {x + z} \right)\hspace{0.75in}\left( {{f_y} = \lambda {g_y}} \right)\label{eq:eq2}\end{equation}\] \[\begin{equation}xy = \lambda \left( {x + y} \right)\hspace{0.75in}\left( {{f_z} = \lambda {g_z}} \right)\label{eq:eq3}\end{equation}\] \[\begin{equation}xy + xz + yz = 32\hspace{0.75in}\left( {g\left( {x,y,z} \right) = 32} \right) \label{eq:eq4} \end{equation}\]There are many ways to solve this system. We’ll solve it in the following way. Let’s multiply equation \(\eqref{eq:eq1}\) by \(x\), equation \(\eqref{eq:eq2}\) by \(y\) and equation \(\eqref{eq:eq3}\) by \(z\). This gives,
\[\begin{equation}xyz = \lambda x\left( {y + z} \right)\label{eq:eq5}\end{equation}\] \[\begin{equation}xyz = \lambda y\left( {x + z} \right)\label{eq:eq6}\end{equation}\] \[\begin{equation}xyz = \lambda z\left( {x + y} \right)\label{eq:eq7}\end{equation}\]Now notice that we can set equations \(\eqref{eq:eq5}\) and \(\eqref{eq:eq6}\) equal. Doing this gives,
\[\begin{align*}\lambda x\left( {y + z} \right) & = \lambda y\left( {x + z} \right)\\ \lambda \left( {xy + xz} \right) - \lambda \left( {yx + yz} \right) &= 0\\ \lambda \left( {xz - yz} \right) & = 0\hspace{0.5in} \Rightarrow \hspace{0.5in}\lambda = 0\,\,\,\,\,\,{\mbox{or}}\,\,\,\,\,xz = yz\end{align*}\]This gave two possibilities. The first, \(\lambda = 0\) is not possible since if this was the case equation \(\eqref{eq:eq1}\) would reduce to
\[yz = 0\hspace{0.5in} \Rightarrow \hspace{0.25in}y = 0\,\,\,{\mbox{or}}\,\,\,z = 0\]Since we are talking about the dimensions of a box neither of these are possible so we can discount \(\lambda = 0\). This leaves the second possibility.
\[xz = yz\]Since we know that \(z \ne 0\) (again since we are talking about the dimensions of a box) we can cancel the \(z\) from both sides. This gives,
\[\begin{equation}x = y\label{eq:eq8}\end{equation}\]Next, let’s set equations \(\eqref{eq:eq6}\) and \(\eqref{eq:eq7}\) equal. Doing this gives,
\[\begin{align*}\lambda y\left( {x + z} \right) & = \lambda z\left( {x + y} \right)\\ \lambda \left( {yx + yz - zx - zy} \right) & = 0\\ \lambda \left( {yx - zx} \right) & = 0\hspace{0.5in} \Rightarrow \hspace{0.5in}\lambda = 0\,\,\,{\mbox{or}}\,\,\,\,yx = zx\end{align*}\]As already discussed we know that \(\lambda = 0\) won’t work and so this leaves,
\[yx = zx\]We can also say that \(x \ne 0\)since we are dealing with the dimensions of a box so we must have,
\[\begin{equation}z = y\label{eq:eq9}\end{equation}\]Plugging equations \(\eqref{eq:eq8}\) and \(\eqref{eq:eq9}\) into equation \(\eqref{eq:eq4}\) we get,
\[{y^2} + {y^2} + {y^2} = 3{y^2} = 32\hspace{0.5in}y = \pm \sqrt {\frac{{32}}{3}} = \pm \,3.266\]However, we know that \(y\) must be positive since we are talking about the dimensions of a box. Therefore, the only solution that makes physical sense here is
\[x = y = z = \,3.266\]So, it looks like we’ve got a cube.
We should be a little careful here. Since we’ve only got one solution we might be tempted to assume that these are the dimensions that will give the largest volume. Anytime we get a single solution we really need to verify that it is a maximum (or minimum if that is what we are looking for).
This is actually pretty simple to do. First, let’s note that the volume at our solution above is,
\[V = f\left( {\sqrt {\frac{{32}}{3}} ,\sqrt {\frac{{32}}{3}} ,\sqrt {\frac{{32}}{3}} } \right) = {\left( {\sqrt {\frac{{32}}{3}} } \right)^3} = 34.8376\]
Now, we know that a maximum of \(f\left( {x,y,z} \right)\) will exist (“proved” that earlier in the solution) and so to verify that that this really is a maximum all we need to do if find another set of dimensions that satisfy our constraint and check the volume. If the volume of this new set of dimensions is smaller that the volume above then we know that our solution does give a maximum.
If, on the other hand, the new set of dimensions give a larger volume we have a problem. We only have a single solution and we know that a maximum exists and the method should generate that maximum. So, in this case, the likely issue is that we will have made a mistake somewhere and we’ll need to go back and find it.
So, let’s find a new set of dimensions for the box. The only thing we need to worry about is that they will satisfy the constraint. Outside of that there aren’t other constraints on the size of the dimensions. So, we can freely pick two values and then use the constraint to determine the third value.
Let’s choose \(x = y = 1\). No reason for these values other than they are “easy” to work with. Plugging these into the constraint gives,
\[1 + z + z = 32\hspace{0.25in} \to \hspace{0.25in}2z = 31\hspace{0.25in} \to \hspace{0.25in}z = \frac{{31}}{2}\]
So, this is a set of dimensions that satisfy the constraint and the volume for this set of dimensions is,
\[V = f\left( {1,1,\frac{{31}}{2}} \right) = \frac{{31}}{2} = 15.5 < 34.8376\]
So, the new dimensions give a smaller volume and so our solution above is, in fact, the dimensions that will give a maximum volume of the box are \(x = y = z = \,3.266\)
Notice that we never actually found values for \(\lambda \) in the above example. This is fairly standard for these kinds of problems. The value of \(\lambda \) isn’t really important to determining if the point is a maximum or a minimum so often we will not bother with finding a value for it. On occasion we will need its value to help solve the system, but even in those cases we won’t use it past finding the point.
This one is going to be a little easier than the previous one since it only has two variables. Also, note that it’s clear from the constraint that region of possible solutions lies on a disk of radius \(\sqrt {136} \) which is a closed and bounded region, \( - \sqrt {136} \le x,y \le \sqrt {136} \), and hence by the Extreme Value Theorem we know that a minimum and maximum value must exist.
Here is the system that we need to solve.
\[\begin{align*}5 & = 2\lambda x\\ - 3 & = 2\lambda y\\ {x^2} + {y^2} & = 136\end{align*}\]Notice that, as with the last example, we can’t have \(\lambda = 0\) since that would not satisfy the first two equations. So, since we know that \(\lambda \ne 0\)we can solve the first two equations for \(x\) and \(y\) respectively. This gives,
\[x = \frac{5}{{2\lambda }}\hspace{0.75in}y = - \frac{3}{{2\lambda }}\]Plugging these into the constraint gives,
\[\frac{{25}}{{4{\lambda ^2}}} + \frac{9}{{4{\lambda ^2}}} = \frac{{17}}{{2{\lambda ^2}}} = 136\]We can solve this for \(\lambda \).
\[{\lambda ^2} = \frac{1}{{16}}\hspace{0.25in} \Rightarrow \hspace{0.25in}\,\,\,\,\,\lambda = \pm \frac{1}{4}\]Now, that we know \(\lambda \) we can find the points that will be potential maximums and/or minimums.
If \(\lambda = - \frac{1}{4}\) we get,
\[x = - 10\hspace{0.75in}y = 6\]and if \(\lambda = \frac{1}{4}\) we get,
\[x = 10\hspace{0.75in}\hspace{0.25in}y = - 6\]To determine if we have maximums or minimums we just need to plug these into the function. Also recall from the discussion at the start of this solution that we know these will be the minimum and maximums because the Extreme Value Theorem tells us that minimums and maximums will exist for this problem.
Here are the minimum and maximum values of the function.
\[\begin{align*}f\left( { - 10,6} \right) & = - 68 & \hspace{0.25in} &{\mbox{Minimum at }}\left( { - 10,6} \right)\\ f\left( {10, - 6} \right) & = 68 & \hspace{0.5in} & {\mbox{Maximum at }}\left( {10, - 6} \right)\end{align*}\]In the first two examples we’ve excluded \(\lambda = 0\) either for physical reasons or because it wouldn’t solve one or more of the equations. Do not always expect this to happen. Sometimes we will be able to automatically exclude a value of \(\lambda \) and sometimes we won’t.
Let’s take a look at another example.
First note that our constraint is a sum of three positive or zero number and it must be 1. Therefore, it is clear that our solution will fall in the range \(0 \le x,y,z \le 1\) and so the solution must lie in a closed and bounded region and so by the Extreme Value Theorem we know that a minimum and maximum value must exist.
Here is the system of equation that we need to solve.
\[\begin{equation}yz = \lambda \label{eq:eq10}\end{equation}\] \[\begin{equation}xz = \lambda \label{eq:eq11}\end{equation}\] \[\begin{equation}xy = \lambda \label{eq:eq12}\end{equation}\] \[\begin{equation}x + y + z = 1 \label{eq:eq13}\end{equation}\]Let’s start this solution process off by noticing that since the first three equations all have \(\lambda \) they are all equal. So, let’s start off by setting equations \(\eqref{eq:eq10}\) and \(\eqref{eq:eq11}\) equal.
\[yz = xz\hspace{0.5in} \Rightarrow \hspace{0.25in}\,\,\,z\left( {y - x} \right) = 0\hspace{0.25in}\,\,\,\,\, \Rightarrow \hspace{0.25in}\,\,\,\,z = 0\,\,\,{\mbox{or}}\,\,\,\,y = x\]So, we’ve got two possibilities here. Let’s start off with by assuming that \(z = 0\). In this case we can see from either equation \(\eqref{eq:eq10}\) or \(\eqref{eq:eq11}\) that we must then have \(\lambda = 0\). From equation \(\eqref{eq:eq12}\) we see that this means that \(xy = 0\). This in turn means that either \(x = 0\) or \(y = 0\).
So, we’ve got two possible cases to deal with there. In each case two of the variables must be zero. Once we know this we can plug into the constraint, equation \(\eqref{eq:eq13}\), to find the remaining value.
\[\begin{align*}z = 0,\,\,x = 0 & : & \Rightarrow \hspace{0.25in}y = 1\\ z = 0,\,\,y = 0 & : & \Rightarrow \hspace{0.25in}x = 1\end{align*}\]So, we’ve got two possible solutions \(\left( {0,1,0} \right)\) and \(\left( {1,0,0} \right)\).
Now let’s go back and take a look at the other possibility, \(y = x\). We also have two possible cases to look at here as well.
This first case is\(x = y = 0\). In this case we can see from the constraint that we must have \(z = 1\) and so we now have a third solution \(\left( {0,0,1} \right)\).
The second case is \(x = y \ne 0\). Let’s set equations \(\eqref{eq:eq11}\) and \(\eqref{eq:eq12}\) equal.
\[xz = xy\hspace{0.5in} \Rightarrow \hspace{0.25in}\,\,\,x\left( {z - y} \right) = 0\hspace{0.25in}\,\,\,\,\, \Rightarrow \hspace{0.25in}\,\,\,\,x = 0\,\,\,{\mbox{or}}\,\,\,z = y\]Now, we’ve already assumed that \(x \ne 0\) and so the only possibility is that \(z = y\). However, this also means that,
\[x = y = z\]Using this in the constraint gives,
\[3x = 1\hspace{0.5in} \Rightarrow \hspace{0.25in}\,\,\,\,\,x = \frac{1}{3}\]So, the next solution is \(\left( {\frac{1}{3},\frac{1}{3},\frac{1}{3}} \right)\).
We got four solutions by setting the first two equations equal.
To completely finish this problem out we should probably set equations \(\eqref{eq:eq10}\) and \(\eqref{eq:eq12}\) equal as well as setting equations \(\eqref{eq:eq11}\) and \(\eqref{eq:eq12}\) equal to see what we get. Doing this gives,
\[\begin{align*}yz & = xy\hspace{0.5in} \Rightarrow \hspace{0.25in}\,\,\,y\left( {z - x} \right) = 0\hspace{0.25in}\,\,\,\,\, \Rightarrow \hspace{0.25in}\,\,\,\,y = 0\,\,\,{\mbox{or}}\,\,\,z = x\\ xz & = xy\hspace{0.5in} \Rightarrow \hspace{0.25in}\,\,\,x\left( {z - y} \right) = 0\hspace{0.25in}\,\,\,\,\, \Rightarrow \hspace{0.25in}\,\,\,\,x = 0\,\,\,{\mbox{or}}\,\,\,z = y\end{align*}\]Both of these are very similar to the first situation that we looked at and we’ll leave it up to you to show that in each of these cases we arrive back at the four solutions that we already found.
So, we have four solutions that we need to check in the function to see whether we have minimums or maximums.
\[\begin{align*}f\left( {0,0,1} \right) & = 0\hspace{0.25in}\,\,\,f\left( {0,1,0} \right) = 0\hspace{0.25in}\,\,\,\,f\left( {1,0,0} \right) = 0 & \hspace{0.25in} & {\mbox{All Minimums}}\\ f\left( {\frac{1}{3},\frac{1}{3},\frac{1}{3}} \right) & = \frac{1}{{27}} & \hspace{0.25in} & {\mbox{Maximum}}\end{align*}\]So, in this case the maximum occurs only once while the minimum occurs three times.
Note as well that we never really used the assumption that \(x,y,z \ge 0\) in the actual solution to the problem. We used it to make sure that we had a closed and bounded region to guarantee we would have absolute extrema. To see why this is important let's take a look at what might happen without this assumption Without this assumption it wouldn’t be too difficult to find points that give both larger and smaller values of the functions. For example.
\[\begin{align*}x = - 100,\,\,y = 100,\,\,z = 1\,\, & :\,\,\,\, - 100 + 100 + 1 = 1\,\,\,\,\,\,\,\,f\left( { - 100,100,1} \right) = - 10000\\ x = - 50,\,\,y = - 50,\,\,z = 101\,\, & :\,\,\,\, - 50 - 50 + 101 = 1\,\,\,\,\,\,\,\,f\left( { - 50, - 50,101} \right) = 252500\end{align*}\]With these examples you can clearly see that it’s not too hard to find points that will give larger and smaller function values. However, all of these examples required negative values of \(x\), \(y\) and/or \(z\) to make sure we satisfy the constraint. By eliminating these we will know that we’ve got minimum and maximum values by the Extreme Value Theorem.
Before we proceed we need to address a quick issue that the last example illustrates about the method of Lagrange Multipliers. We found the absolute minimum and maximum to the function. However, what we did not find is all the locations for the absolute minimum. For example, assuming \(x,y,z\ge 0\), consider the following sets of points.
\[\begin{array}{ll} \left( 0,y,z \right) & \text{where}\,\,\,\,y+z=1 \\ \left( x,0,z \right) & \text{where}\,\,\,\,x+z=1 \\ \left( x,y,0 \right) & \text{where}\,\,\,\,x+y=1 \\ \end{array}\]Every point in this set of points will satisfy the constraint from the problem and in every case the function will evaluate to zero and so also give the absolute minimum.
So, what is going on? Recall from the previous section that we had to check both the critical points and the boundaries to make sure we had the absolute extrema. The same was true in Calculus I. We had to check both critical points and end points of the interval to make sure we had the absolute extrema.
It turns out that we really need to do the same thing here if we want to know that we’ve found all the locations of the absolute extrema. The method of Lagrange multipliers will find the absolute extrema, it just might not find all the locations of them as the method does not take the end points of variables ranges into account (note that we might luck into some of these points but we can’t guarantee that).
So, after going through the Lagrange Multiplier method we should then ask what happens at the end points of our variable ranges. For the example that means looking at what happens if \(x=0\), \(y=0\), \(z=0\), \(x=1\), \(y=1\), and \(z=1\). In the first three cases we get the points listed above that do happen to also give the absolute minimum. For the later three cases we can see that if one of the variables are 1 the other two must be zero (to meet the constraint) and those were actually found in the example. Sometimes that will happen and sometimes it won’t.
In the case of this example the end points of each of the variable ranges gave absolute extrema but there is no reason to expect that to happen every time. In Example 2 above, for example, the end points of the ranges for the variables do not give absolute extrema (we’ll let you verify this).
The moral of this is that if we want to know that we have every location of the absolute extrema for a particular problem we should also check the end points of any variable ranges that we might have. If all we are interested in is the value of the absolute extrema then there is no reason to do this.
Okay, it’s time to move on to a slightly different topic. To this point we’ve only looked at constraints that were equations. We can also have constraints that are inequalities. The process for these types of problems is nearly identical to what we’ve been doing in this section to this point. The main difference between the two types of problems is that we will also need to find all the critical points that satisfy the inequality in the constraint and check these in the function when we check the values we found using Lagrange Multipliers.
Let’s work an example to see how these kinds of problems work.
Note that the constraint here is the inequality for the disk. Because this is a closed and bounded region the Extreme Value Theorem tells us that a minimum and maximum value must exist.
The first step is to find all the critical points that are in the disk (i.e. satisfy the constraint). This is easy enough to do for this problem. Here are the two first order partial derivatives.
\[\begin{align*}{f_x} & = 8x & & \Rightarrow \hspace{0.25in}\,\,\,8x = 0\hspace{0.25in} \Rightarrow \hspace{0.25in}\,\,\,\,\,\,x = 0\\ {f_y} & = 20y & & \Rightarrow \hspace{0.25in}20y = 0\hspace{0.25in} \Rightarrow \hspace{0.25in}\,\,\,\,\,\,y = 0\end{align*}\]So, the only critical point is \(\left( {0,0} \right)\) and it does satisfy the inequality.
At this point we proceed with Lagrange Multipliers and we treat the constraint as an equality instead of the inequality. We only need to deal with the inequality when finding the critical points.
So, here is the system of equations that we need to solve.
\[\begin{align*}8x & = 2\lambda x\\ 20y & = 2\lambda y\\ {x^2} + {y^2} & = 4\end{align*}\]From the first equation we get,
\[2x\left( {4 - \lambda } \right) = 0\hspace{0.5in} \Rightarrow \hspace{0.25in}\,\,\,\,\,x = 0\,\,\,\,{\mbox{or}}\,\,\,\,\,\lambda = 4\]If we have \(x = 0\) then the constraint gives us \(y = \pm \,2\).
If we have \(\lambda = 4\) the second equation gives us,
\[20y = 8y\hspace{0.25in} \Rightarrow \hspace{0.25in}\,\,\,y = 0\]The constraint then tells us that \(x = \pm \,2\).
If we’d performed a similar analysis on the second equation we would arrive at the same points.
So, Lagrange Multipliers gives us four points to check :\(\left( {0,2} \right)\), \(\left( {0, - 2} \right)\), \(\left( {2,0} \right)\), and \(\left( { - 2,0} \right)\).
To find the maximum and minimum we need to simply plug these four points along with the critical point in the function.
\[\begin{align*}f\left( {0,0} \right) & = 0 & \hspace{0.25in} & {\mbox{Minimum}}\\ f\left( {2,0} \right) & = f\left( { - 2,0} \right) = 16 & &\\ f\left( {0,2} \right) & = f\left( {0, - 2} \right) = 40 & \hspace{0.25in} & {\mbox{Maximum}}\end{align*}\]In this case, the minimum was interior to the disk and the maximum was on the boundary of the disk.
The final topic that we need to discuss in this section is what to do if we have more than one constraint. We will look only at two constraints, but we can naturally extend the work here to more than two constraints.
We want to optimize \(f\left( {x,y,z} \right)\) subject to the constraints \(g\left( {x,y,z} \right) = c\) and \(h\left( {x,y,z} \right) = k\). The system that we need to solve in this case is,
\[\begin{align*}\nabla f\left( {x,y,z} \right) & = \lambda \nabla g\left( {x,y,z} \right) + \mu \nabla h\left( {x,y,z} \right)\\ g\left( {x,y,z} \right) & = c\\ h\left( {x,y,z} \right) & = k\end{align*}\]So, in this case we get two Lagrange Multipliers. Also, note that the first equation really is three equations as we saw in the previous examples. Let’s see an example of this kind of optimization problem.
Verifying that we will have a minimum and maximum value here is a little trickier. Clearly, because of the second constraint we’ve got to have \( - 1 \le x,y \le 1\). With this in mind there must also be a set of limits on \(z\) in order to make sure that the first constraint is met. If one really wanted to determine that range you could find the minimum and maximum values of \(2x - y\) subject to \({x^2} + {y^2} = 1\) and you could then use this to determine the minimum and maximum values of \(z\). We won’t do that here. The point is only to acknowledge that once again the possible solutions must lie in a closed and bounded region and so minimum and maximum values must exist by the Extreme Value Theorem.
Here is the system of equations that we need to solve.
\[\begin{equation}0 = 2\lambda + 2\mu x\hspace{0.5in}\left( {{f_x} = \lambda {g_x} + \mu {h_x}} \right)\label{eq:eq14}\end{equation}\] \[\begin{equation}4 = - \lambda + 2\mu y\hspace{0.5in}\left( {{f_y} = \lambda {g_y} + \mu {h_y}} \right)\label{eq:eq15}\end{equation}\] \[\begin{equation} - 2 = - \lambda \hspace{0.5in}\left( {{f_z} = \lambda {g_z} + \mu {h_z}} \right)\label{eq:eq16}\end{equation}\] \[\begin{equation}2x - y - z = 2 \label{eq:eq17}\end{equation}\] \[\begin{equation}{x^2} + {y^2} = 1 \label{eq:eq18}\end{equation}\]First, let’s notice that from equation \(\eqref{eq:eq16}\) we get \(\lambda = 2\). Plugging this into equation \(\eqref{eq:eq14}\) and equation \(\eqref{eq:eq15}\) and solving for \(x\) and \(y\) respectively gives,
\[\begin{align*}0 & = 4 + 2\mu x & \hspace{0.1in} & \Rightarrow \hspace{0.5in}x = - \frac{2}{\mu }\\ 4 & = - 2 + 2\mu y & \hspace{0.1in} & \Rightarrow \hspace{0.5in}y = \frac{3}{\mu }\end{align*}\]Now, plug these into equation \(\eqref{eq:eq18}\).
\[\frac{4}{{{\mu ^2}}} + \frac{9}{{{\mu ^2}}} = \frac{{13}}{{{\mu ^2}}} = 1\hspace{0.25in}\,\,\,\,\,\,\, \Rightarrow \hspace{0.5in}\,\mu = \pm \,\sqrt {13} \]So, we have two cases to look at here. First, let’s see what we get when \(\mu = \sqrt {13} \). In this case we know that,
\[x = - \frac{2}{{\sqrt {13} }}\hspace{0.5in}y = \frac{3}{{\sqrt {13} }}\]Plugging these into equation \(\eqref{eq:eq17}\) gives,
\[ - \frac{4}{{\sqrt {13} }} - \frac{3}{{\sqrt {13} }} - z = 2\hspace{0.5in} \Rightarrow \hspace{0.5in}z = - 2 - \frac{7}{{\sqrt {13} }}\]So, we’ve got one solution.
Let’s now see what we get if we take \(\mu = - \sqrt {13} \). Here we have,
\[x = \frac{2}{{\sqrt {13} }}\hspace{0.5in}y = - \frac{3}{{\sqrt {13} }}\]Plugging these into equation \(\eqref{eq:eq17}\) gives,
\[\frac{4}{{\sqrt {13} }} + \frac{3}{{\sqrt {13} }} - z = 2\hspace{0.5in} \Rightarrow \hspace{0.5in}z = - 2 + \frac{7}{{\sqrt {13} }}\]and there’s a second solution.
Now all that we need to is check the two solutions in the function to see which is the maximum and which is the minimum.
\[\begin{align*}f\left( { - \frac{2}{{\sqrt {13} }},\frac{3}{{\sqrt {13} }}, - 2 - \frac{7}{{\sqrt {13} }}} \right) & = 4 + \frac{{26}}{{\sqrt {13} }} = 11.2111\\ f\left( {\frac{2}{{\sqrt {13} }}, - \frac{3}{{\sqrt {13} }}, - 2 + \frac{7}{{\sqrt {13} }}} \right) & = 4 - \frac{{26}}{{\sqrt {13} }} = - 3.2111\end{align*}\]So, we have a maximum at \(\left( { - \frac{2}{{\sqrt {13} }},\frac{3}{{\sqrt {13} }}, - 2 - \frac{7}{{\sqrt {13} }}} \right)\) and a minimum at \(\left( {\frac{2}{{\sqrt {13} }}, - \frac{3}{{\sqrt {13} }}, - 2 + \frac{7}{{\sqrt {13} }}} \right)\).